Implementation of Proximal Soil Sensing, Data Fusion and Machine Learning to Improve Phosphorus Management at a Field Scale
In the context of a rapid increase in phosphorus (P) fertilizers prices, new techniques are needed for geospatial predictions of soil P for improved P fertilizer management, while increasing farmer profitability and reducing environmental concerns. One of the biggest issues in site-specific phosphorus management is the substantial spatial variability in plant available P across fields. This leads to an expensive and laborious process for accurate mapping soil P using a traditional soil sampling and laboratory analysis approach. To overcome this barrier, emerging sensing and data interpretation technologies should be employed to accurately assess spatial heterogeneity of P within fields, and to help farmers optimize mineral P fertilization recommendations.
In this study, we applied machine learning algorithms and novel data fusion concepts to analyze integrated high-density spatial data layers related to the potential P availability to plants. Machine learning algorithms were used to evaluate the relative importance of different auxiliary soil properties at predicting plant-available P. These variables were used for modeling P spatial distribution. High-density data mining techniques and various sensor data fusion algorithms and optimization techniques were used to predict P spatial distribution and identify site-specific management zones. Supervised machine learning algorithms such as Support Vector Machine was used, along with sensor data fusion.
Auxiliary data to predict available P included high-density apparent soil electrical conductivity (ECa), gamma-ray spectrometry, high-resolution topography as well as soil test data from a field in Southwestern Ontario, Canada. Spatial maps were prepared using elevation data based on a Real-Time Kinematic (RTK) global navigation satellite systems (GNSS) receiver, DUALEM-21S sensing, a gamma-ray (SoilOptix) spectrometer and laboratory analysis of soil samples. An ATV was used to collect on-the-go measurements using this proximal soil sensing equipment.
The machine learning models successfully predicted P and generated high resolution maps showing P status zones and recommendation maps at the local level. The results of supervised regressions and reclassifications modeling represent a robust decision tool for phosphorus precision nutrient management and variable rate technology. Regression machine learning models were turned into classification models. Results of this study show that radial basis kernel trick SVM regressor trained and validated with 10 fold repeated cross validation performed the best. In fact, a cost value equal to 1, sigma=0.08 and epsilon=0.1, provides an R-squared of 0.53 and 0.54, an RMSE of 8.79 and 8.98 for SVM K-fold cross validation and repeated cross-validation respectively. Therefore, the choice of repeated cross-validation slightly improved our available P spatial predictions.
Environmental sensor covariate fusion combined with spatial machine learning algorithms is a useful tool to help farmers save P fertilizer and preserve environmental resources through understanding the available P spatial variability across the study area. Future research should focus on combining prediction results based on different machine learning algorithms and integrate them with traditional regression techniques.
Speaker
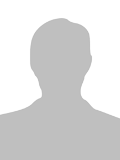