Monitoring Corn (Zea mays) Yield using Sentinel-2 and Machine Learning for Precision Agriculture Applications
Currently, there is a growing demand to apply precision agriculture (PA) management practices at agricultural fields expecting more efficient and more profitable management. One of PA principal components for site-specific management is crop yield monitoring which varies temporally between seasons and spatially within-field. In this study, we investigated the possibility of monitoring within-field variability of corn grain yield in a 22ha field located in Ferarra, North Italy. Archived yield data for 2016, 2017 and 2018 seasons were correlated with different vegetation indices derived from Sentinel-2 satellite images at different crop growth stages. Yield data was filtered to remove field boundaries and other outliers to maintain yield maps accuracy. A total of 34 cloud-free satellite images (6 images for 2016, 14 for 2017 and 14 for 2018 season) were analysed and vegetation indices such as Green Normalized Difference Vegetation Index (GNDVI) were calculated. Vegetation indices of each season were compared with the actual corn yield map for the same season and models accuracy metrics were calculated for each index and image date. Furthermore, different machine learning techniques such as random forests, support vector machine and multiple regression were applied to develop the most accurate model out of all sentinel-2 bands and available yield data. In addition, accuracy metrics such as error metrics and coefficient of determination were calculated and all developed models were applied to all images to examine each model applicability. Results of this work are as follows: Firstly, GNDVI was the most accurate vegetation index to monitor within-field variability of corn yield with an R2 value of 0.48 and showed the same trend for all studied seasons. Secondly, crop age of 120 days after sowing (R4-R6) showed the best results for corn yield prediction which is during summer in Italy (July to August) with less cloud probability. Thirdly, random forests provided the highest performance for investigating within-field corn yield variability with R2 value over 0.5. This study provides a tool for monitoring within-field variability that could be applied for archived satellite images to provide farmers with their historical yield spatial variability.
Speaker
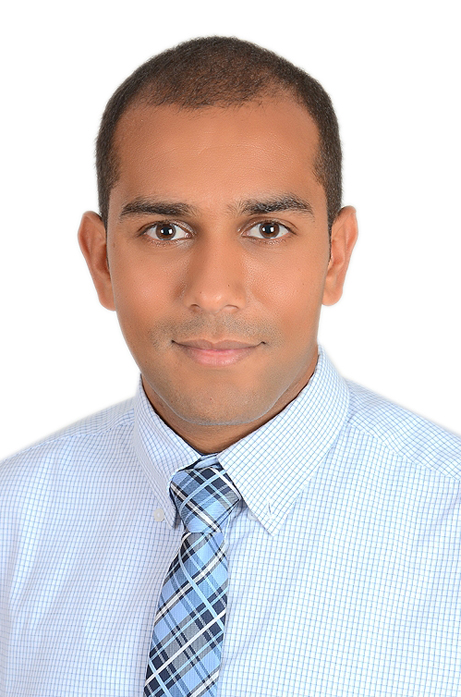